AI and Open Data: a crucial combination
Unlocking the potential of Artificial Intelligence
AI and data: a crucial combination
Artificial Intelligence (AI) technology has the capacity to extract deeper insights from datasets than other techniques. Examples of AI are: speech recognition, natural language, processing, chatbots or voice bots. To get AI applications to work, big sets of high quality (open) data are necessary. But what requirements does this data have to meet? To answer this question, we need to look more closely at what requirements data need to have to enable successful AI applications.
Use of Open Data for AI
Access to (open) data can unlock the potential of AI applications. An example of an AI project worth noting shows how big amounts of Open Data are used at a police agency that focuses on crime prevention. Based on occurrences of crimes (data) and their frequency, the algorithm developed patterns to identify 'hotspots', areas where certain crimes are likely to occur in the future. These 'hotspots' are defined geographic areas within which the algorithm can make specific predictions as to the type of crime that could take place and when it is most likely to occur. Underlying these predictions are certain assumptions and patterns, such as that criminals often operate within the same area for long periods of time. This project was very successful, reducing burglaries by 33% and violent crimes by 21% in the city where it was applied.
The ingredients to let AI to function properly
AI systems need Open Data to function. To function properly, significant 1) data volume, 2) data variety and 3) data veracity (the truthfulness of data) are crucial. In traditional data analysis, when bad data is discovered, one can exclude the bad data and start over. However, this way of data cleansing cannot be done on a larger scale. Bad data cannot be detected or 'pulled out' of the system that easily. AI techniques draw conclusions from large masses of data and at some point, it becomes impossible to determine on which data elements these predictions are based. Often, when bad data is detected, the whole learning process starts again from the beginning, which is time- and cost-intensive.
For this reason, besides the V's 'volume' and 'variety', 'veracity' is needed to detect whether the data is of good quality. Veracity refers to the truthfulness of the data. There are several questions to answer to find out whether the data is 'truthful':
- Why & Who: Data from a reliable source implies better accuracy than a random online poll. Data is sometimes collected, or even fabricated, to serve an agenda. Therefore, a user of data should establish the credibility of the data source, and for what purpose it was collected.
- Where: Data is often geographically or culturally biased. Consumer data collected in one area of the world may not be representative of consumers 200 kilometres further. In addition to this, the interpretation of data can differ: when we objectively measure data, such as temperatures, the interpretation of that data can differ: what is considered cold or warm?
- When: Most data are linked to time in some way that it might be a time series, or it can be a snapshot from a specific period. Out-of-date data should be omitted. However, when using AI over a longer time span, data can become old or obsolete during the process. "Machine unlearning" will be needed to get rid of data that is no longer valid.
- How: It is important to understand the 'core' of how the data of interest was collected. Domain knowledge is of the essence here. For instance, when collecting consumer data, we can fall back on the decades old methods of market research. Answers on an ill-constructed questionnaire will certainly render poor quality data.
- What: If you use certain data, you want to know what your data is about, but before you can do that, you should know what surrounds the numbers. Humans can sometimes detect bad data, for instance because it looks illogical. However, AI does not have this form of common sense and tends to take all data for true.
Together with the three 'V's, Open Data can play a pivotal role in achieving benefits through the use of AI. Open Data is data that is made available by (public) organisations, businesses and individuals for anyone to access, use and share. When data is not open, data cannot be re-used for other purposes, such as AI. Access to open data will 'unlock' the potential of data-hungry machine AI applications.
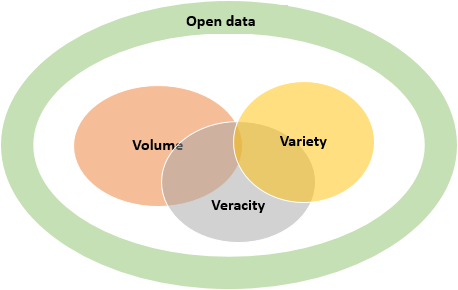
Figure 1 Open Data and AI
How to achieve data quality?
Data quality requires an integral approach to make sure that people, processes and systems within organisations meet the demands to achieve continuous data quality. Three factors are important:
- Define roles and responsibilities to employees for efficient data management;
- Describe processes for data quality assurance;
- Invest in sufficient technology (software and IT architecture) to support employees.
With the right software, people and rules in place, organisations can ensure that the organisational data system detects errors and delivers valuable new insights by making use of AI.
The future of AI?
Data is essential for AI as algorithms in these systems need large quantities of high-quality data to perform properly and to develop further by 'learning'. The continuous promotion of data quality within organisations when using (open) data for AI is therefore essential to gain reliable insights. An important focus for (public) organisations is to make data openly available where possible to let other organisations (or people within their own organisation) use the data. Increasing access to high-quality data volumes is key to unleashing the potential of AI and to allow innovation to flourish.